Stuck between AGI and RAG? Fret not, we’re here to unravel the mysteries of artificial intelligence for you.
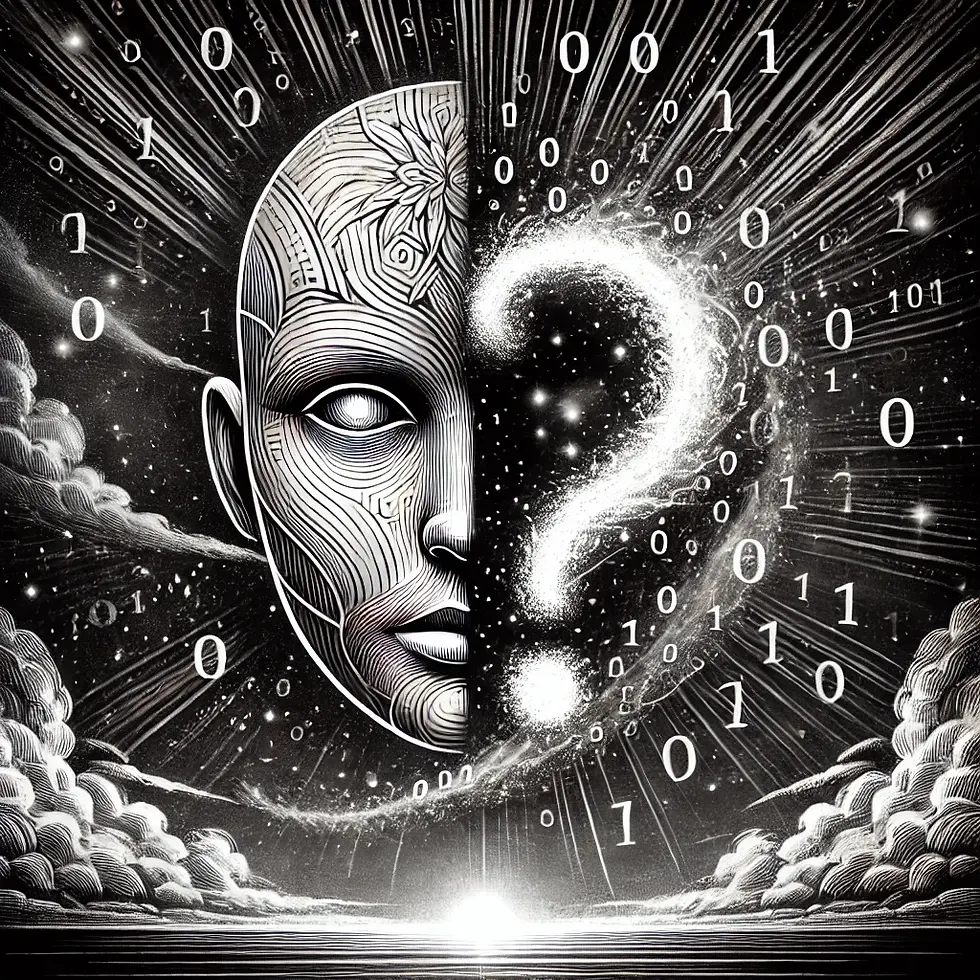
Artificial intelligence is the shiny new toy in the tech world. Every company and their CEO is buzzing about how they’re revolutionizing their products with AI. Yet, with every press release and keynote speech, they toss around so much jargon it feels like we need a PhD just to understand what’s going on. If you’re feeling a bit lost in the AI fog, fear not — this cheat sheet is your guiding light through the dense and sometimes confusing forest of AI terminology.
What Exactly is AI?
Let’s start with the basics. Artificial Intelligence (AI) is technically a field of computer science dedicated to making machines that can think like humans. But let’s be honest, it’s also become a bit of a buzzword. Depending on who you ask — or what a company wants you to think — AI can mean anything from sophisticated algorithms to your smart fridge that remembers you like oat milk over regular.
Companies like Google and OpenAI have been talking up their AI capabilities for years, with AI models powering their products behind the scenes. Meanwhile, Meta’s Mark Zuckerberg loves to refer to individual chatbots as “AI,” adding to the mix of how the term gets used (and abused). As more businesses jump on the AI bandwagon, the definitions and jargon can get even muddier.
Breaking Down the Buzzwords: Your Artificial Intelligence Glossary
To help you sound like you know what you’re talking about at your next tech-savvy dinner party, here’s a breakdown of the most common AI terms floating around the ether.
1. Machine Learning: The Backbone of AI
Machine Learning (ML) is where the magic happens. These systems are trained on data so they can make predictions about new information. Think of it like teaching a dog tricks: you show it enough examples (data), and it learns how to sit, roll over, or recognize your face.
2. Artificial General Intelligence (AGI): The Sci-Fi Dream
Artificial General Intelligence (AGI) is the stuff of science fiction — AI that is as smart as, or smarter than, a human. It’s the dream (or nightmare, depending on who you ask) where machines become our intellectual equals. Think Skynet from The Terminator, but hopefully less homicidal.
3. Generative AI: Creating Content Out of Thin Air
Generative AI can create new text, images, code, and more. Ever seen a funny (or terrifyingly off) image from an AI tool? That’s generative AI at work. Models like OpenAI’s GPT and Google’s Gemini are prime examples, using vast datasets to learn how to mimic human creativity — sometimes with hilarious results.
4. Hallucinations: When AI Gets it Very, Very Wrong
No, we’re not talking about psychedelic trips. Hallucinations in AI are when these tools start making things up — confidently. They produce answers or images that seem accurate but are, in fact, complete nonsense. So next time your AI chatbot tells you that Albert Einstein invented the iPhone, just remember — it’s having a hallucination.
5. Bias: When AI Picks Up Human Flaws
Because AI tools are created by humans, they can inherit our biases. A famous study by MIT Media Lab’s Joy Buolamwini and DAIR’s Timnit Gebru showed that facial recognition software had higher error rates with darker-skinned women. Just like humans, if AI is trained on biased data, it will produce biased results.
Demystifying AI Models: What Are They, Exactly?
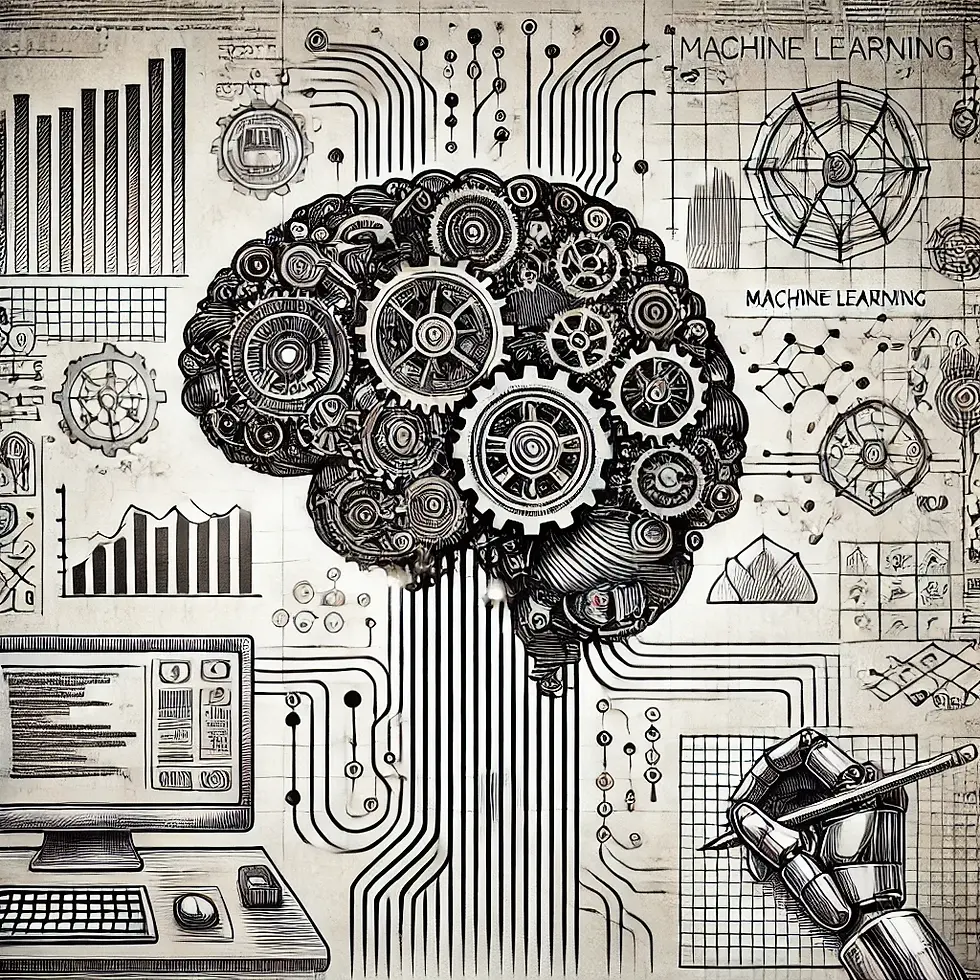
Now that you’ve got a handle on some of the basic terms, you’re probably wondering, “What’s all this talk about models?” In the AI world, a model is a fancy name for a trained system that can perform tasks or make decisions.
Large Language Models (LLMs): The Chatty AI Tools
LLMs are a type of AI model that can process and generate text. If you’ve ever chatted with a bot that’s almost too real, that’s likely an LLM in action. OpenAI’s ChatGPT is one example, using deep learning to understand and generate text that feels eerily human.
Diffusion Models: AI with an Artistic Flair
Diffusion models are behind those cool AI-generated images you see all over the internet. They start by adding noise to images and then learning to reverse the process. Think of it like un-blurring a foggy picture — but done by a computer that’s never seen a sunset but knows exactly how to paint one.
Foundation Models: The Jack of All Trades
Foundation models are trained on massive datasets and serve as a versatile foundation for various applications. OpenAI’s GPT, Google’s Gemini, Meta’s Llama, and Anthropic’s Claude all fit this mold. They’re the Swiss Army knives of AI, capable of a wide range of tasks without needing specific training for each one.
How Do AI Models Learn?
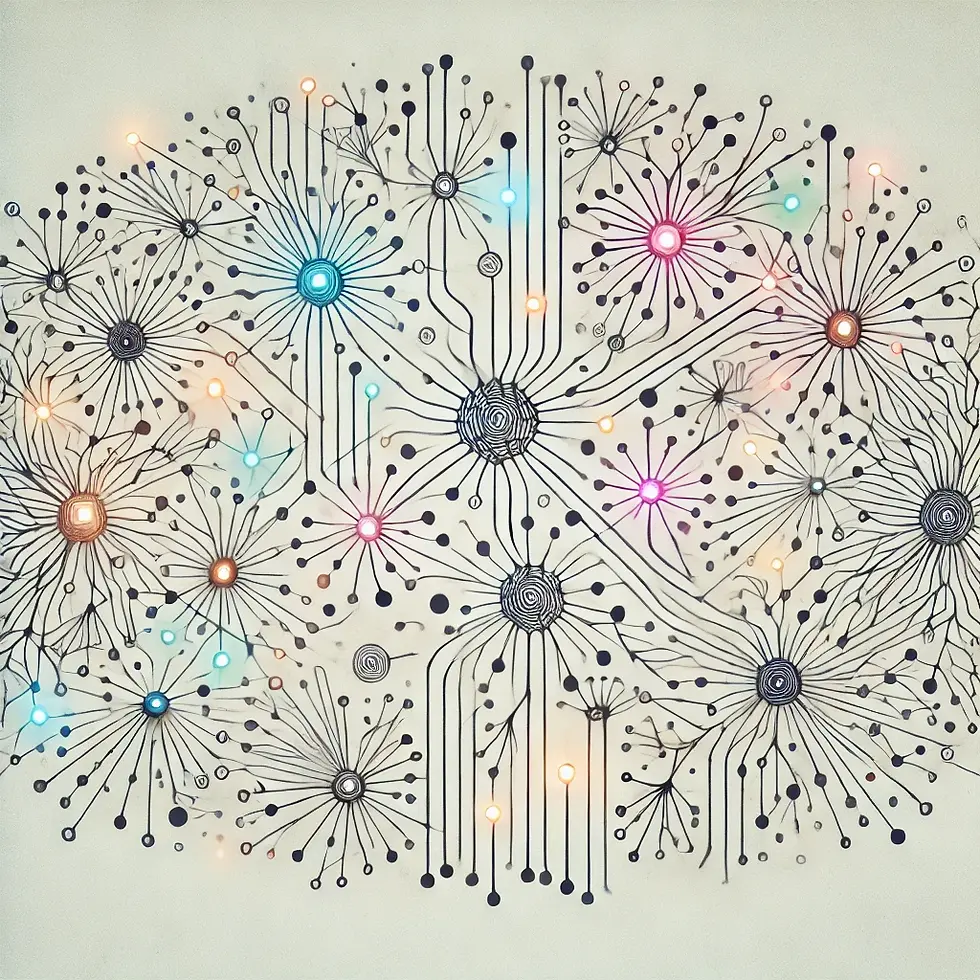
Glad you asked! AI models get all their smarts from a process called training. This involves feeding them lots of data and letting them learn patterns, just like cramming for a final exam. The bigger the data (like all of Wikipedia), the smarter the model (theoretically).
Parameters: The Secret Sauce of AI Learning
During training, AI models adjust their parameters — think of these as the settings on a machine learning model that dictate how it processes input and generates output. The more parameters, the more sophisticated the model (again, in theory).
Navigating the AI Hardware Landscape
Of course, all this AI wizardry requires some serious hardware. Enter the Nvidia H100 chip — the favorite child of AI training. It’s what’s powering most of the advanced AI training happening today. Then you have Neural Processing Units (NPUs) in your devices that help with day-to-day AI tasks like video call enhancements. And finally, TOPS — or trillion operations per second — a term companies love to throw around to show off how powerful their chips are.
The Usual Suspects: Key Players in the AI Game
Here are some of the main players behind today’s AI boom:
OpenAI / ChatGPT: The company behind the chatbot sensation that made AI a household topic.
Microsoft / Copilot: Integrating AI into all its products through a partnership with OpenAI.
Google / Gemini: Google’s AI umbrella that covers everything from search to email.
Meta / Llama: Meta’s open-source model designed to democratize AI.
Apple / Apple Intelligence: Bringing AI capabilities into its ecosystem, including Siri.
Anthropic / Claude: A new player in the AI field, backed by Amazon and Google investments.
xAI / Grok: Elon Musk’s AI venture, looking to disrupt the AI landscape.
Perplexity: Known for its AI-powered search engine, under scrutiny for its practices.
Hugging Face: The go-to platform for finding AI models and datasets.
Conclusion: So, What Does All This Mean for You?
Navigating the AI landscape can feel like walking through a dense jungle, filled with jargon and buzzwords. But understanding the basics — from machine learning to foundation models — can help demystify this ever-evolving field. Whether you’re a tech enthusiast or just someone trying to keep up with the conversation, we hope this cheat sheet helps you feel a bit more confident the next time AI comes up at the dinner table.
We hope you now understand these concepts better and learned something useful today.
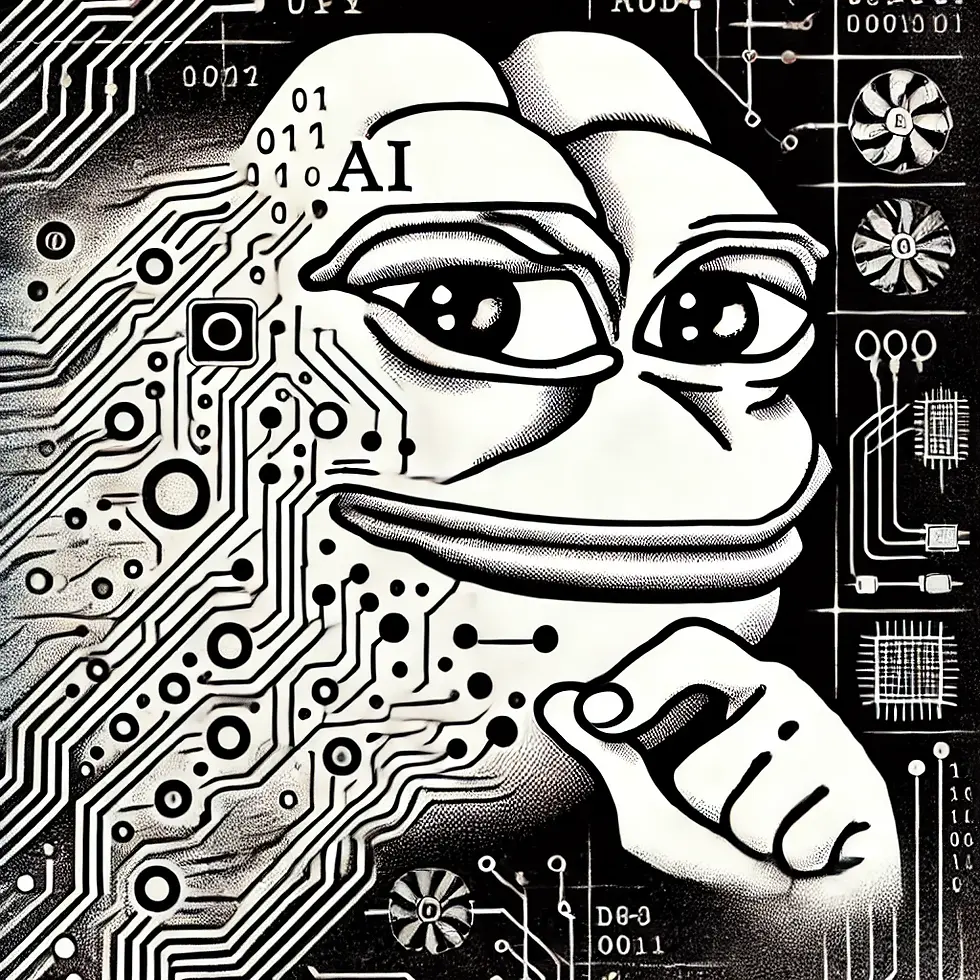
Comments